Computer scientist investigates fairness in healthcare and imaging diagnostics
Jessica Werb
For Laleh Seyyed-Kalantari, assistant professor in the Department of Electrical Engineering and Computer Science in the Lassonde School of Engineering, conducting research on the fairness of AI in healthcare and imaging diagnostics is personal.
While working on research in developing optimization algorithms, Seyyed-Kalantari’s life was derailed by a medical misdiagnosis that took two years to resolve. It was an agonizing experience, but it also inspired her to find a way to prevent the same thing from happening to others.
“I saw the impact of having an under-diagnosis in my personal life,” she says. “I remember wishing that my research could help patients reduce pain.”
Seyyed-Kalantari found a way to do just that during her post-doctoral fellowships at the Vector Institute and the University of Toronto, where she began investigating inaccuracies in AI diagnostics—work that she continues to pursue at York.
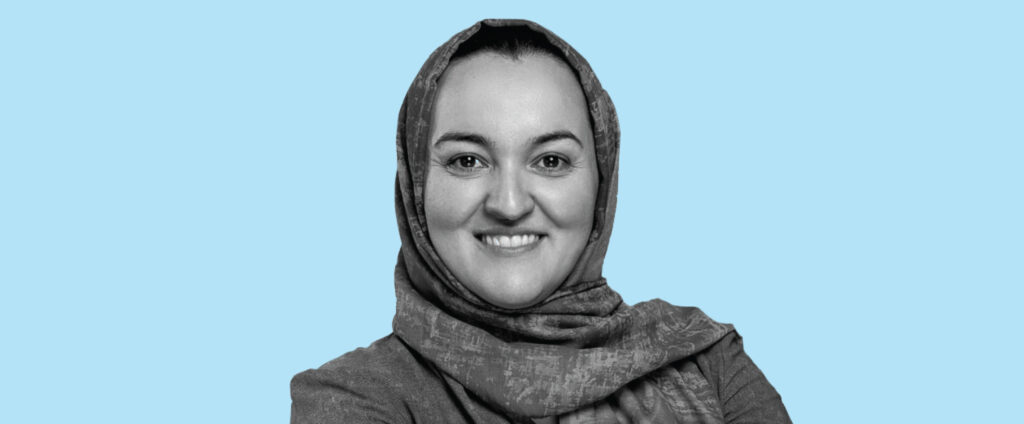
And she’s uncovered some troubling findings that have caught even her by surprise.
In a paper published in Nature Medicine, Seyyed-Kalantari, as the lead author, examined data from multiple sources in the U.S. and discovered that AI-driven screening tools of chest X-rays had a concerning rate of under-diagnosis among underserved patient populations.
“Historically vulnerable subpopulations—for example, Black, Hispanic, female and low-income patients—are suffering more from AI mistakes in these algorithms compared to other sub-populations,”
she explains, noting that under-diagnoses are particularly harmful. “Upon deployment of such AI models, these patients were wrongly diagnosed as healthy. That means they may not have received any treatment in a timely manner and were sent home without further assessments.”
Even radiologists were taken aback, she added. “They said that when reviewing patient results, they don’t know anything about the patient’s race. They are sitting in a dark room reviewing the images and asking themselves ‘How can we be unfair to a patient that we have never seen?’”
So, Seyyed-Kalantari and her multi-disciplinary, multi-institutional team delved further, looking into whether AI could determine the race of a patient from X-rays, CT scans and mammographic images alone. The results, published in The Lancet Digital Health, were astonishing.
“With a very high accuracy, AI models can determine the race of the patient by just looking at medical images,”
says Seyyed-Kalantari. “Everybody was surprised, and it was alarming. We don't know what AI is doing with this information. While we find that AI models can detect the race of patients, we find at the same time that AI is behaving against some races.”
The question now is: how is it happening? And what are the repercussions?
Seyyed-Kalantari is actively looking for answers as she delves deeper into her work at Lassonde. In the meantime, she urges caution in embedding AI into healthcare.
“Some AI algorithms have received FDA approval in the U.S. for applications in radiology. But to the best of my knowledge, they haven’t proven that the
algorithms are fair,” she warns.
“If we’re deploying these algorithms and using them for disease diagnoses -and we’re not sure if they’re fair or not -this could harm some groups in our society.”
This concern underscores why research like Seyyed-Kalantari’s is so important, and how further research in this field can help ensure that the healthcare of tomorrow is truly fair and equitable.
Read more
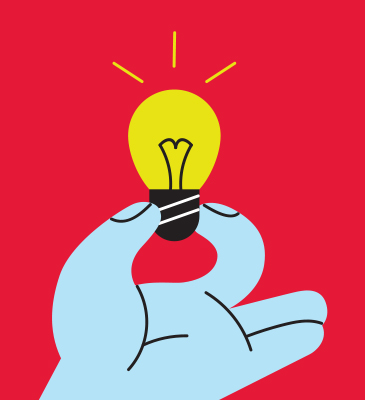
Meet York U’s Commercialization Fellows
Four budding researchers aim to bring innovative products to market
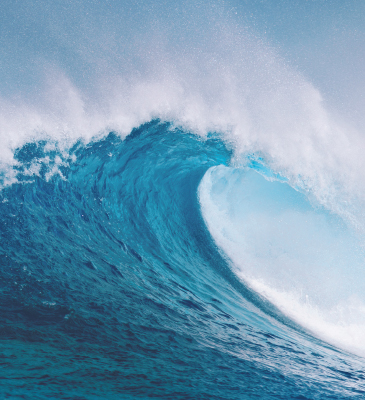
New organized research unit of early success, impact
A new Organized Research Unit (ORU) in its infancy but is already driving positive change
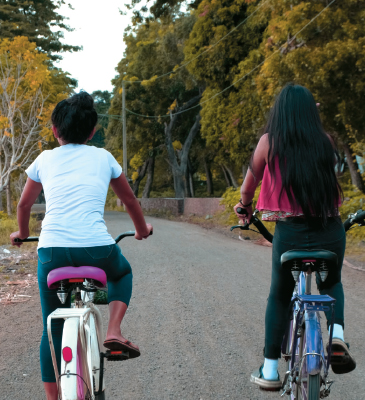
York health researcher brings a trauma-informed lens to use sport for good
How women find empowerment and independence on two wheels
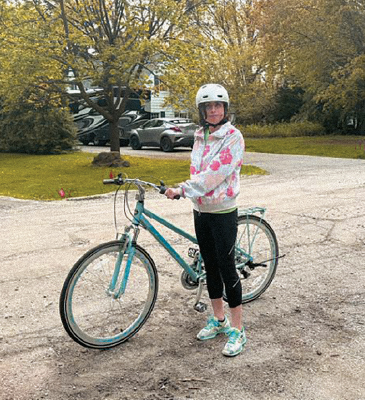
York engineers develop mobile app to improve the safety of city cycling
Using tech to help take the yikes out of bikes