Post
Published on November 25, 2024
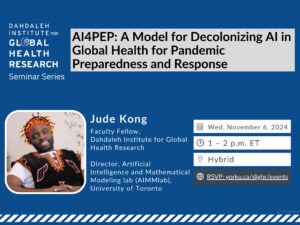
On November 6, Dahdaleh faculty fellow Professor Jude Kong presented an overview of his work in developing and implementing decolonized Artificial Intelligence (AI) frameworks, particularly within public health contexts across Africa and the Global South. He began by detailing the structure and reach of his network, which spans 21 countries, emphasizing the value of consistent communication and collaboration among members. This community centered approach which contributes to knowledge sharing and the adoption of effective strategies tailored to local needs.
Professor Kong introduced the three foundational pillars of his work which includes ensuring timely and reliable health data, strengthening healthcare systems and promoting the inclusion and equity of vulnerable groups. He highlighted the proactive nature of AI driven solutions, such as early warning systems to prevent disease outbreaks, which are crucial for neglected communities that often receive attention only when crises escalate.
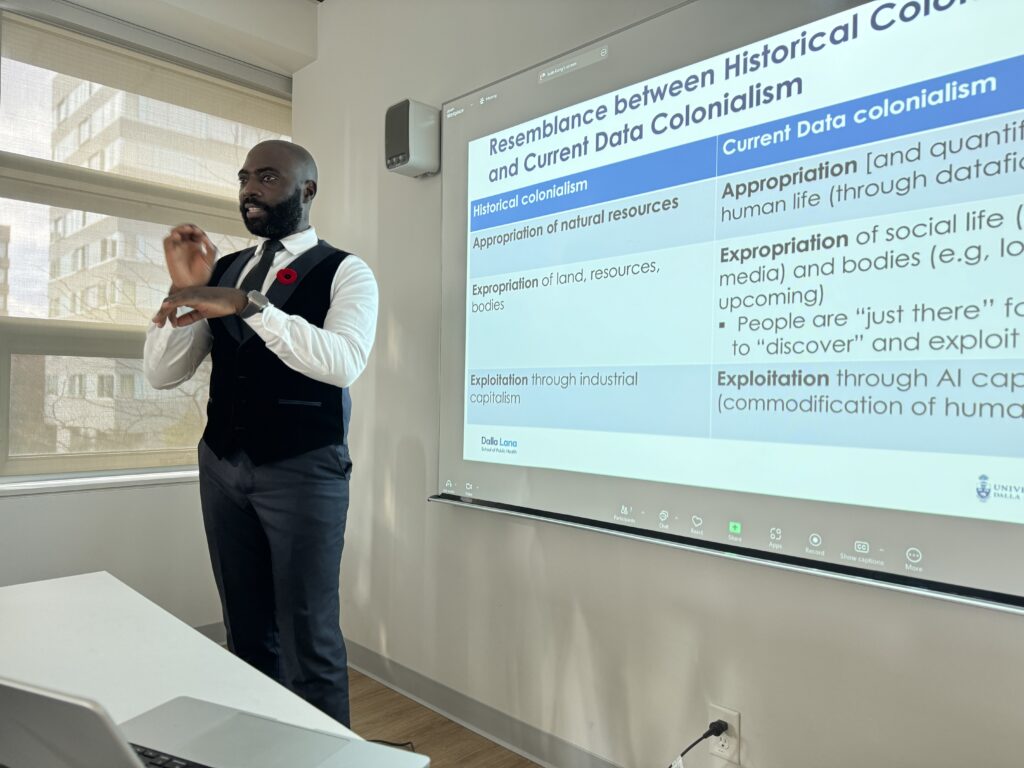
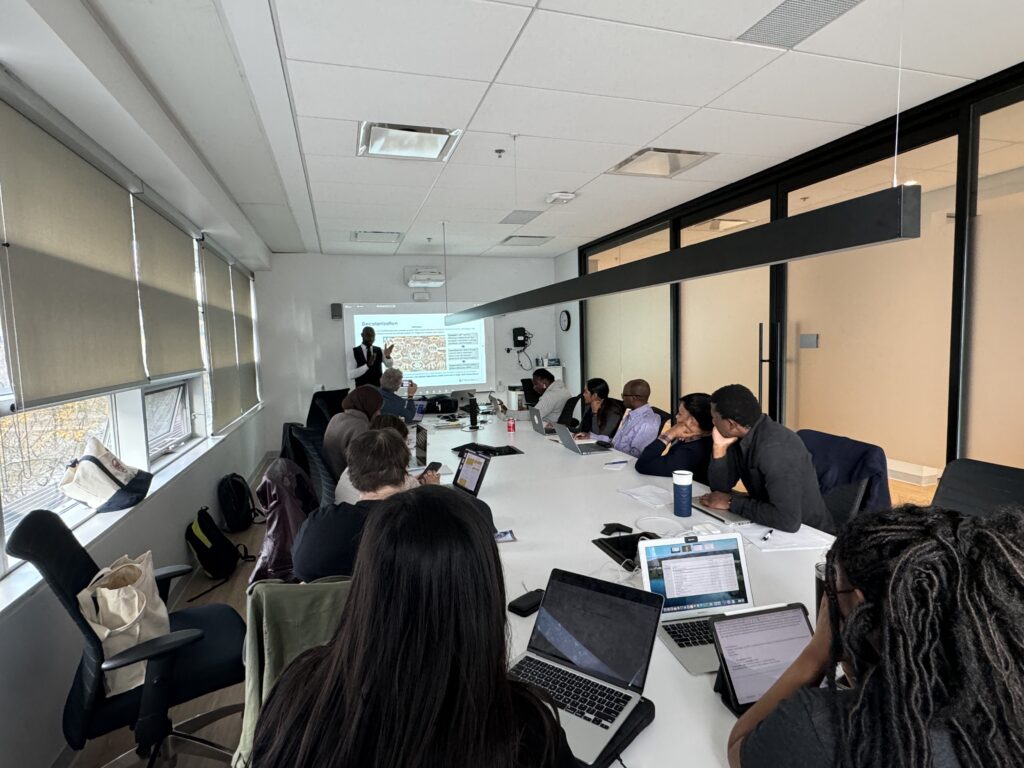
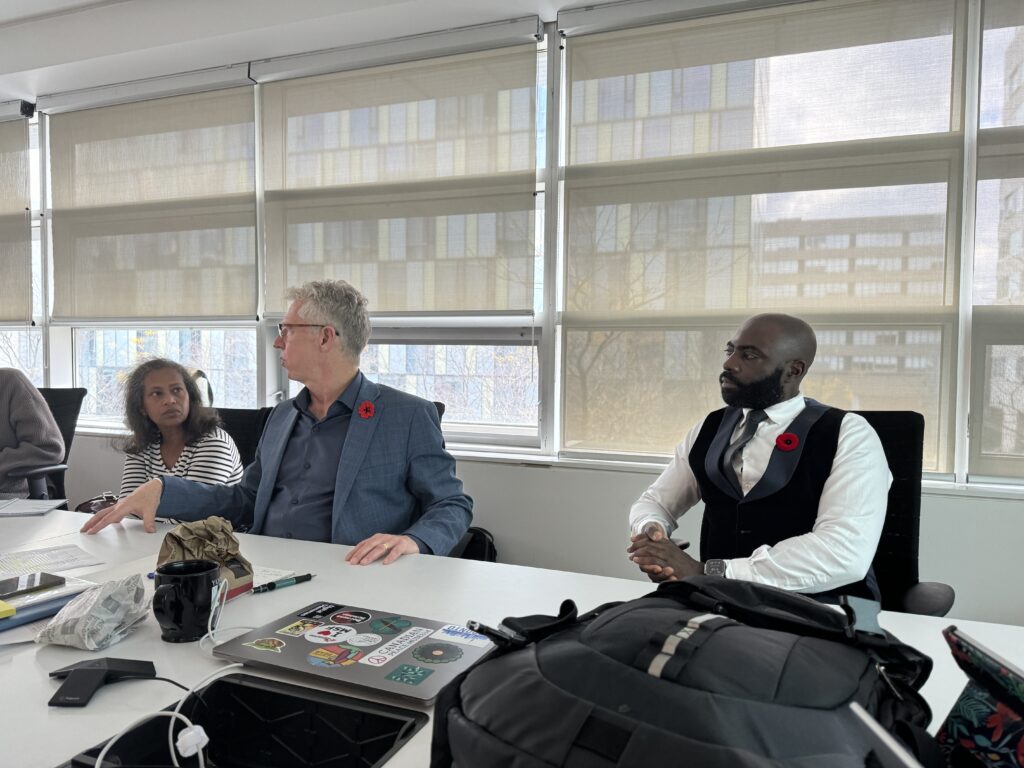
One of the core concepts Professor Kong explored was the decolonization of AI. He defined this as dismantling historical colonial structures that influence technology and emphasized the need for locally relevant and co-created solutions. This requires engaging communities throughout the AI development process, from data collection to model validation. He stressed the importance of understanding community needs, collaborating with local stakeholders and ensuring solutions are culturally and contextually appropriate to avoid stigmatization or ineffectiveness.
Addressing Bias and Decolonization in the Machine Learning (ML) Pipeline: A Stage-Wise Framework
To systematically reduce bias and ensure that ML solutions are inclusive, equitable, fair, and aligned with principles of decolonization, each stage of the ML pipeline must incorporate specific responsible practices. Below is a structured framework for achieving these goals:
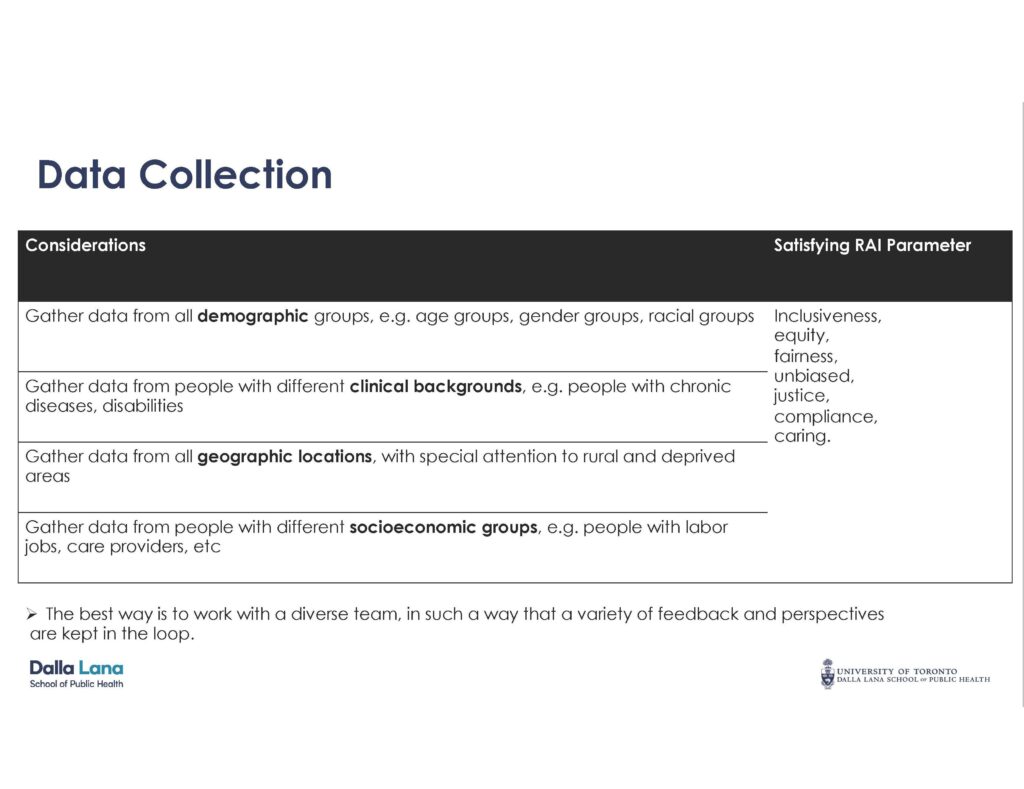
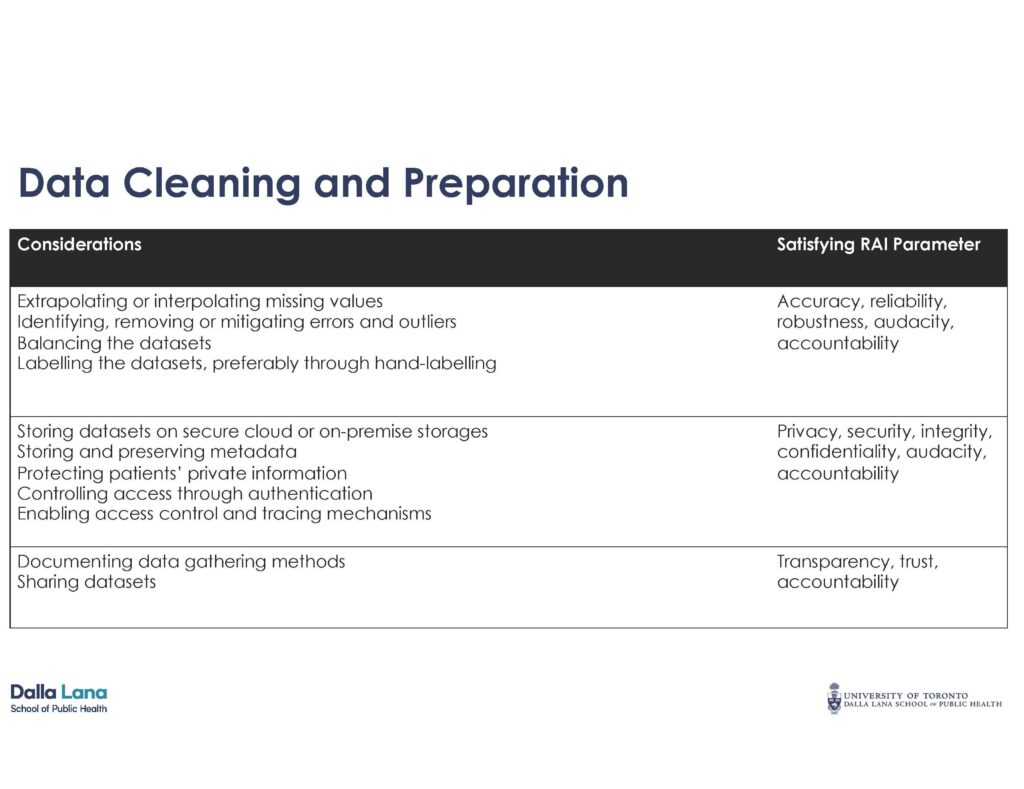
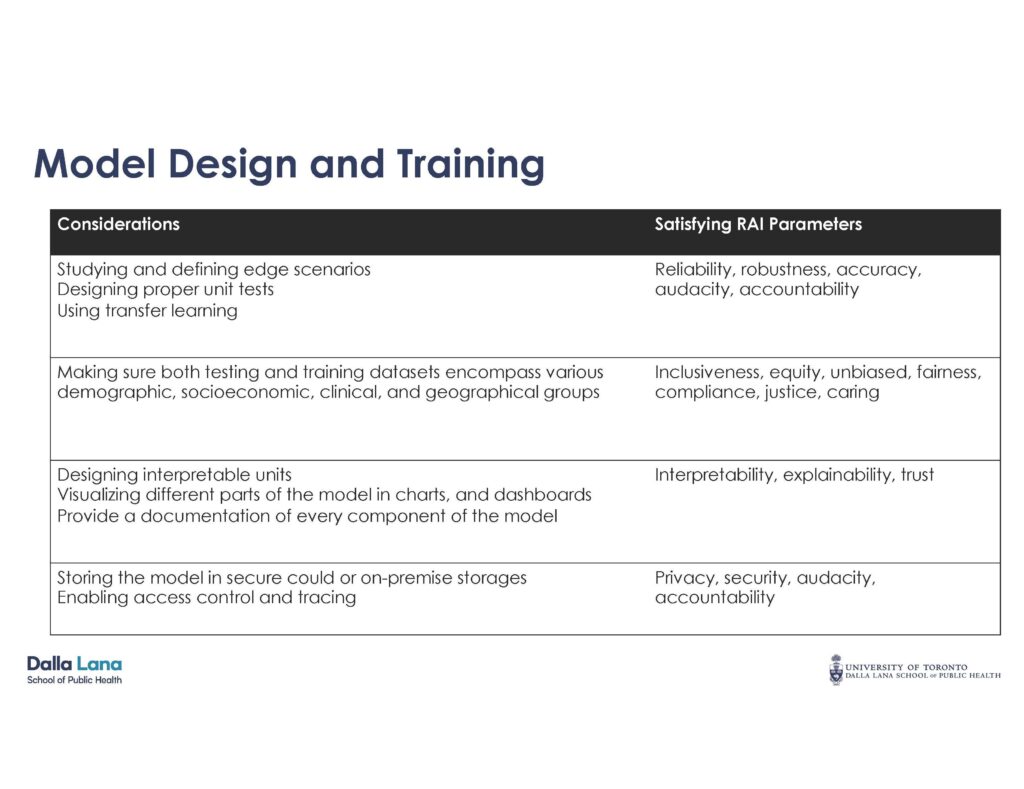
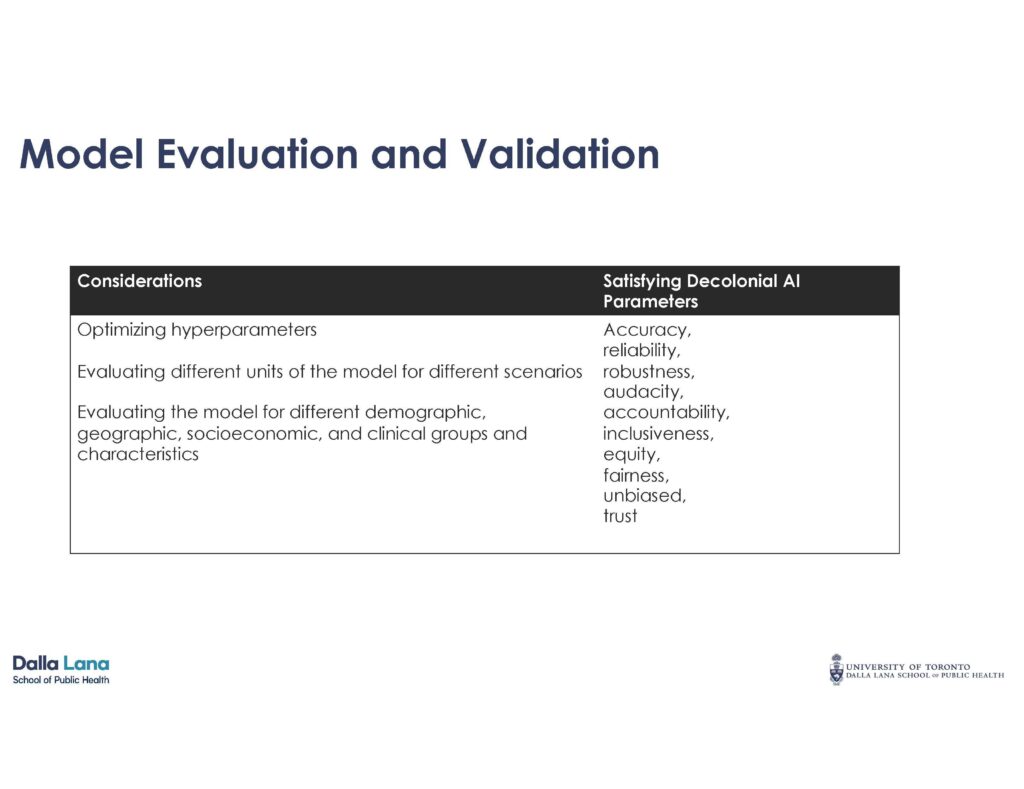
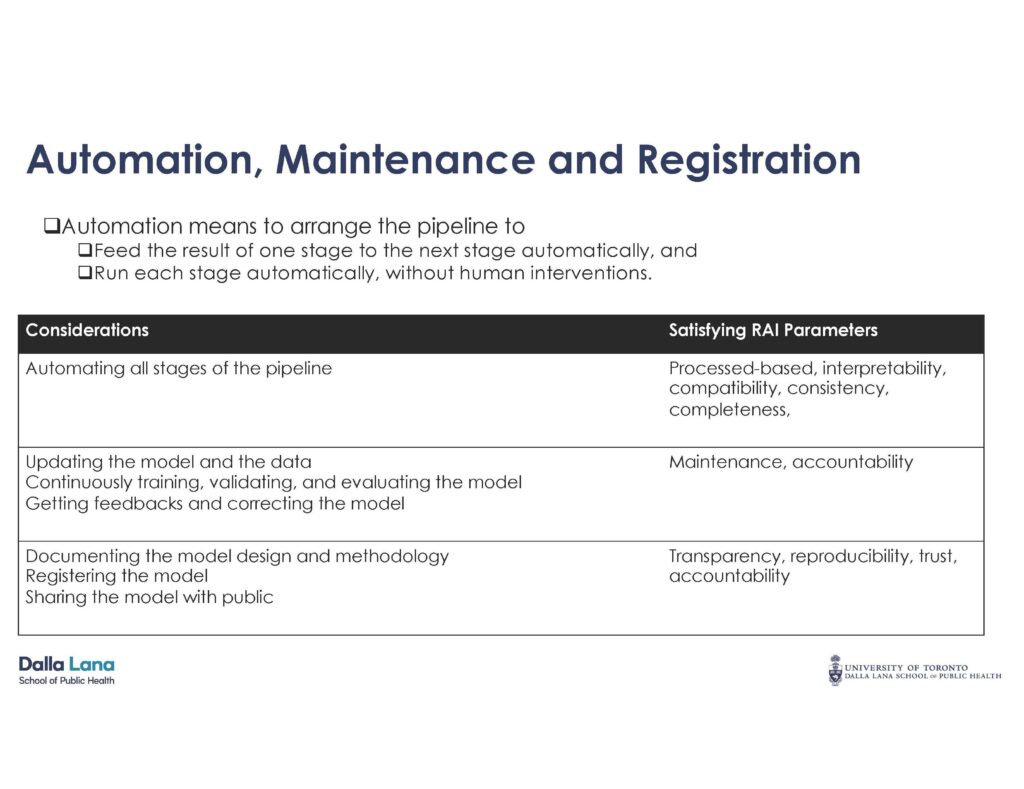
Professor Kong explained the practical application of these principles with case studies, such as tools for early detection of acute paralysis in Ethiopia, a fake news detection system adopted in Brazil and mosquito surveillance technology in Ghana. He concluded by discussing sustainability, noting the important role of governments in adopting and funding these technologies. Through this seminar, Professor Kong presented the significance of a decolonized approach to AI, where community involvement and localized data are central to creating sustainable and impactful solutions in public health.
Watch the seminar presentation below: https://www.youtube.com/watch?v=RFSj062ZorI
Connect with Jude Kong.
Themes | Global Health & Humanitarianism |
Status | Active |
Related Work | |
Updates |
N/A
|
People |
You may also be interested in...
Advancing Social Science Research at UN Multi-stakeholder Hearings on Tuberculosis
Despite commendable advances in new Tuberculosis (TB) diagnostics and more safe and effective TB treatments, as depicted in the latest Global TB Report, 10.6 million people fell ill, and 1.6 million people died of TB ...Read more about this Post
GEHLab Interns Present at 2024 CUGH Conference in Los Angeles, California
From March 7th to March 10th, 2024, two Global and Environmental Health Lab members attended the 15th annual Consortium of Universities for Global Health (CUGH) Conference held in Los Angeles, California. Dahdaleh global health alumna Mirianna Georges and ...Read more about this Post
Recap – Climate Change Threatens to Cause Greater Resource Insecurity, Greater Poverty, and Poor Health Outcomes
On February 1, over 40 attendees were introduced to Godfred Boateng's research programs – he discussed some examples of current global environmental changes that have destabilized the earth's climate and threaten to cause resource insecurity, ...Read more about this Post