For more information on our course offerings, please go to York Course Website.
This course invites participants to reflect on the practice of teaching mathematics in light of research, theory, and their own experiences. It examines how teachers draw on mathematical and pedagogical knowledge in their work. A specialised mathematics background is not a prerequisite.
This course invites participants to reflect on the practice of teaching mathematics in light of research, theory, and their own experiences. It examines how teachers draw on mathematical and pedagogical knowledge in their work. A specialised mathematics background is not a prerequisite.
Quantitative Research Methods in Education/Same as Mathematics & Statistics 5910 3.0 Calendar course description: This course discusses theoretical, practical and ethical considerations, decisions and processes involved in conducting quantitative research in education. It explores the research process from formulating research questions, study design, sampling, data collection, analysis and interpretation, to communicating research processes and findings.
Presently there are no research methods courses that focus on the particular needs of the students interested in doing research in mathematics education. This course is intended as a companion to the course in qualitative and quantitative methods.
Presently there are no research methods courses that focus on the particular needs of the students interested in doing research in mathematics education. This course is intended as a companion to the course in qualitative and quantitative methods.
Grading of a Survey Paper will follow the format of grading of other courses in the programme (i.e. A+, A, etc.). A student will normally be required to present his/her paper in a student Colloquium. The final grade will be partially dependent on the student's performance during such a presentation.
Grading of a Survey Paper will follow the format of grading of other courses in the programme (i.e. A+, A, etc.). A student will normally be required to present his/her paper in a student Colloquium. The final grade will be partially dependent on the student's performance during such a presentation.
Grading of a Survey Paper will follow the format of grading of other courses in the programme (i.e. A+, A, etc.). A student will normally be required to present his/her paper in a student Colloquium. The final grade will be partially dependent on the student's performance during such a presentation.
Grading of a Survey Paper will follow the format of grading of other courses in the programme (i.e. A+, A, etc.). A student will normally be required to present his/her paper in a student Colloquium. The final grade will be partially dependent on the student's performance during such a presentation.
Students are required to present two one-hour seminars under faculty supervision and to attend other students' presentations. Written reports on the seminars are also required. The topics can be chosen from any field of mathematics but must be distinct from the material of the student's coursework, thesis or survey paper.
Students are required to present two one-hour seminars under faculty supervision and to attend other students' presentations. Written reports on the seminars are also required. The topics can be chosen from any field of mathematics but must be distinct from the material of the student's coursework, thesis or survey paper.
Students are required to present two one-hour seminars under faculty supervision and to attend other students' presentations. Written reports on the seminars are also required. The topics can be chosen from any field of mathematics but must be distinct from the material of the student's coursework, thesis or survey paper.
Students are required to present two one-hour seminars under faculty supervision and to attend other students' presentations. Written reports on the seminars are also required. The topics can be chosen from any field of mathematics but must be distinct from the material of the student's coursework, thesis or survey paper.
Group theory and representation theory, preliminary notions in ring (Euclidian domain, principal ideal domain and polynomial rings), Grobner bases with some emphasis on algorithmic aspects and computational geometry, modules over a PID (linear algebra). Prerequisite: equivalent of undergraduate second-year linear algebra and some basic notions in algebra (group, ring), or permission of the Instructor.
Introduction to category theory, additional ring and module theory, introduction to algebraic geometry, field theory and Galois theorems. Prerequisite: GS/MATH 6121 3.00 or permission of the Instructor.
Introduction to category theory, additional ring and module theory, introduction to algebraic geometry, field theory and Galois theorems. Prerequisite: GS/MATH 6121 3.00 or permission of the Instructor.
This course is an introduction to the mathematics of public-key cryptography. Topics include finite fields, DLP-based cryptography, elliptic curve cryptography, signature schemes, classical and quantum attacks on these cryptosystems, and the mathematics of post-quantum cryptography (code and lattice-based cryptosystems). A familiarity with undergraduate algebra is required.
s-algebras, generating classes, finite and s-finite measures on general spaces, constructing measures from outer measures, Caratheodory extension theorem, Jordan decomposition into variations. Hahn decomposition, measurable functions, Lusin's theorem, Lebesgue integral on general spaces, convergence theorems in an abstract setting, Radon-Nikodym theorem, Riesz representation theorem, Maharam's classification of measure algebras, product spaces, Fubini's theorem, non-measurable sets, Lebesgue density theorem.
s-algebras, generating classes, finite and s-finite measures on general spaces, constructing measures from outer measures, Caratheodory extension theorem, Jordan decomposition into variations. Hahn decomposition, measurable functions, Lusin's theorem, Lebesgue integral on general spaces, convergence theorems in an abstract setting, Radon-Nikodym theorem, Riesz representation theorem, Maharam's classification of measure algebras, product spaces, Fubini's theorem, non-measurable sets, Lebesgue density theorem.
Complex differentiation and integration; Cauchy's integral formula; Taylor series; the residue theorem; Rouche's theorem; the Riemann mapping theorem, conformal mappings, harmonic functions and boundary value problems, Schwarz's reflection principle, infinite products and Mittag-Leffler expansions.
Fourier transforms, homogeneous distributions, singular integrals, spherical harmonics, pseudo-differential operators, fundamental solutions and parametrices of partial differential operators.
An introduction to Banach and Hilbert spaces together with bounded linear functionals and operators on these spaces. Topics include: the Hahn-Banach theorem, representation of dual spaces, the uniform boundedness principle, the open mapping and closed graph theorems, compact operators.
Filters, nets, separation, continuity, operations on topological spaces, function spaces, compactness, Stone-Cech compactification, connectedness, metric spaces, completeness, metrization theorems, covering properties, cardinal functions, dimension theory.
A systematic presentation of many statistical concepts and techniques for the analysis of time series data. The core topics include time dependence and randomness, trend, seasonality and error, stationary process, ARMA and ARIMA processes, multivariate time series and state-space models. Integrated with the undergraduate course SC/MATH 4130B 3.00.
This course provides students with an introduction to the statistical methods for analyzing sensored data which are common in medical research, industrial life-testing and related fields. Topics include accelerated life models, proportional hazards model, time dependent covariates. Integrated with the undergraduate course Mathematics & Statistics 4130K 3.0. Prerequisites: York undergraduate Mathematics 3033 3.0 and 3132 3.0 or equivalents, or permission of the instructor.
This course provides students with an introduction to the statistical methods for analyzing sensored data which are common in medical research, industrial life-testing and related fields. Topics include accelerated life models, proportional hazards model, time dependent covariates. Integrated with the undergraduate course Mathematics & Statistics 4130K 3.0. Prerequisites: York undergraduate Mathematics 3033 3.0 and 3132 3.0 or equivalents, or permission of the instructor.
Offers a comprehensive introduction to statistical learning theory including most widely used methods such as classification trees, support vector machine and model averaging. Prerequisites: GS/MATH 6620 3.00 and GS/MATH 6630 3.00 or equivalents or permission of the Instructor.
This course serves as an introduction to data science from the perspective of statistics. The course begins with an introduction of relevant computational and analytical tools necessary to analyze large data sets (with the understanding that the tools which are most relevant change over time) including Python, R and SAS. The course will then focus on visualisation tools and exploratory data analysis, high dimensional statistical tools such as LASSO and tensor analysis, as well as causality and propensity scores. The students will learn how these tools are implemented on large data sets through case studies.
Numerical methods for solving ordinary differential equations, optimization problems: steepest descents, conjugate gradient methods, approximation theory: least squares, orthogonal polynomials, Chebyshev and Fourier approximation, Padé approximation. Prerequisite: SC/MATH 2270 3.00; SC/MATH 3242 3.00 or LE/EECS 3122 3.00 or their equivalents.
Provides a rigorous treatment of numerical methods for the solutions of ordinary and partial differential equations.
Provides a rigorous treatment of numerical methods for the solutions of ordinary and partial differential equations.
Probability models and discrete time stochastic processes; Brownian motion; Filtrations, conditional expectations, martingales; Stochastic integrals, Ito's formula; Stochastic differential equations; Diffusions, Kolmogorov equation; Girsanov Formula; Black Scholes.
Probability models and discrete time stochastic processes; Brownian motion; Filtrations, conditional expectations, martingales; Stochastic integrals, Ito's formula; Stochastic differential equations; Diffusions, Kolmogorov equation; Girsanov Formula; Black Scholes.
Introduction to Partial Differential Equations; basic properties of the diffusion equation; Finite difference methods; explicit methods; implicit methods including Crank Nicholson; Free boundary problems; variational inequalities; Lattice Methods.
Introduction to Partial Differential Equations; basic properties of the diffusion equation; Finite difference methods; explicit methods; implicit methods including Crank Nicholson; Free boundary problems; variational inequalities; Lattice Methods.
This introductory course on machine learning is aimed at graduate students who are going to work with data scientists in the finance industry. The course explains the most popular machine learning algorithms used by data scientists, which enables the students to assess their strength and weakness for a particular application and work productively with data scientists. Algorithms are illustrated with different data sets from the finance industry and others in EXCEL and Python.
This introductory course on machine learning is aimed at graduate students who are going to work with data scientists in the finance industry. The course explains the most popular machine learning algorithms used by data scientists, which enables the students to assess their strength and weakness for a particular application and work productively with data scientists. Algorithms are illustrated with different data sets from the finance industry and others in EXCEL and Python.
This graduate course prepares students for today's data-driven finance industry, where the volume and velocity of financial data are the foundation of trading, pricing, forecasting, and risk management. Python is the choice of programming language in this course as it has just emerged as one of the major strategic technology platforms. The course will start with an immerse introduction of Python at a fast speed suitable for graduate students and will spend majority of time on financial data: how to programmatically access, process, analyze, and utilize financial data offline and online.
This course will cover the basic tools required to critically read modelling papers and to develop and use models as research tools. Models of infectious disease; threshold conditions for epidemic outbreaks, the basic reproductive rate of a disease; vaccination strategies to control infection. Emphasis will be placed on setting up and utilizing mathematical models to understand infectious disease processes and to evaluate potential control strategies. This course will provide an opportunity for students who are interested in using mathematical modelling techniques to study the transmission dynamics of infectious disease on a population level. The course will help to develop modelling, analytical and computational expertise in both continuous time and discrete time dynamical models for the study of infectious diseases. Prerequisites: Atkinson/Arts/Science Mathematics & Statistics 2270 3.0: Differential equations or equivalent and some numerical skills or equivalent and familiarity with Maple or Matlab or Mathematica
This course will cover the basic tools required to critically read modelling papers and to develop and use models as research tools. Models of infectious disease; threshold conditions for epidemic outbreaks, the basic reproductive rate of a disease; vaccination strategies to control infection. Emphasis will be placed on setting up and utilizing mathematical models to understand infectious disease processes and to evaluate potential control strategies. This course will provide an opportunity for students who are interested in using mathematical modelling techniques to study the transmission dynamics of infectious disease on a population level. The course will help to develop modelling, analytical and computational expertise in both continuous time and discrete time dynamical models for the study of infectious diseases. Prerequisites: Atkinson/Arts/Science Mathematics & Statistics 2270 3.0: Differential equations or equivalent and some numerical skills or equivalent and familiarity with Maple or Matlab or Mathematica
This course introduces perturbative methods as techniques for finding approximate solutions to mathematical problems. The course begins with approximating roots to polynomials before exploring applications in linear algebra, integrals, and differential equations. This course is integrated with MATH 4940. Prerequisites: Students should have familiarity with calculus, linear algebra, and differential equations.
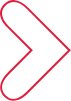
Learn More
The Graduate Program in Mathematics and Statistics at York is an exciting environment to pursue innovative, socially engaging, career-ready education. Contact our Graduate Program Assistant to learn more.