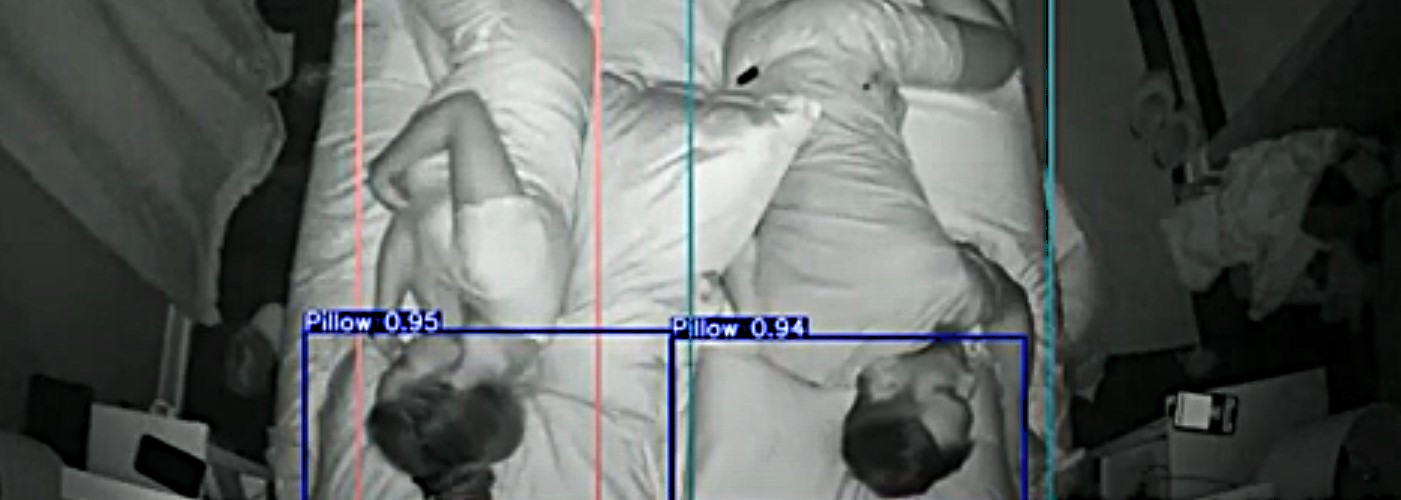
AI could help solve questions about health of sleeping on back after 28-weeks pregnancy
The importance of sleep for improving brain function, mood, cardiovascular health, metabolic health and staving off dementia has emerged as a hot topic, but for pregnant women, sleep position may also prove critical for giving birth to a healthy child.
Although there is a known association between back sleeping after 28 weeks of pregnancy and low-weight infants and stillbirth, the casual proof is difficult to ascertain, and some experts doubt the connection altogether. However, York University’s Elham Dolatabadi and team have developed a computer vision tool that can identify various sleep postures during pregnancy and potentially lead to more definitive answers in the future.
An assistant professor in York’s School of Health Policy and Management, Faculty of Health, and a member of York’s Centre for AI and Society and Connected Minds, Dolatabadi is interested in developing artificial intelligence and machine learning solutions to health issues. She spent much of her early years developing sensing technologies, also called ambient intelligence, and was in search of a real-world issue when her former student Allan Kember of the University of Toronto, now an obstetrician/gynecologist at Mount Sinai Hospital, suggested maternal sleeping posture.
“These types of technologies that I’ve worked on can be embedded in the environment and the maternal sleeping posture and poor infant outcomes is a modifiable risk factor,” she says.
Along with the Vector Institute, where she was formerly an applied scientist and health lead, Dolatabadi and team have come out with the second version of an AI tool designed to monitor the sleeping positions of pregnant women throughout the night.
“We've developed a vision-based tool that automatically detects the sleep postures of pregnant women using video recordings. This tool is part of a larger initiative aimed at creating unobtrusive and affordable health sensing technologies,” says Dolatabadi. “What’s remarkable is that the tool can accurately detect common sleep postures, such as side and back sleeping, even in real-world settings with blankets, pillows, and more than one person in bed.”
The research team used video from an observational, four-night, home sleep apnea study with 15pregnant participants and 13 bed partners along with controlled-setting video recordings from a previous in-home, simulation study where 26 participants simulated a series of 12 pre-defined body postures. Pregnant participants were between 28- and 40-weeks gestation from across Canada.
The data was combined and used to train and test the tool, which was able to detect 13 pre-defined sleeping positions, including sitting. Although it did better at detecting side, back and sitting positions, it learned to distinguish the anatomy and physiology of a pregnant person compared to their non-pregnant partner – or, in one case, the pets which were also in the bed – as well as pelvis position, says Dolatabadi, adding these were some of the complexities involved in training the AI tool.

Sleep positions included supine pelvis with left or right thorax tilts, supine thorax with right or left pelvic tilts, and prone. It was also able to detect pillows and blankets.
In real-world applications, the tool will allow for the study of pregnant women in a natural setting, providing more, higher quality and more accurate information than is currently available as it won’t be relying on self reports and someone’s memory of their sleeping position.
As Dolatabadi says, “This technology has the potential to answer how sleep positions may affect pregnancy outcomes, including factors like baby size and stillbirth risk, and solve the controversy and uncertainty about the association between the supine sleeping posture and outcomes.”
In the future, the team plans to refine the tool with plans to make it multi-modal. This would involve training it to monitor and predict heart rate and other vitals using just a camera or phone. It is possible to predict things like heart rate using vision tools without the need for more invasive monitoring sensors, she says.
This would not only provide more robust data to assess the risk of sleep position but also to predict the risk of sleep apnea without people having to go to a sleep clinic.
“The more we can infuse into the model, the better outcome that we can get,” says Dolatabadi.
Going forward, Dolatabadi is interested in further evaluating generative AI to ensure models are not propagating or creating inequities in health-care environments.
“It’s important to evaluate health AI applications as they are susceptible to biases and harm, whether it’s medical diagnostics or administrative billing for hospital services. There are models of this nature that have been integrated into health-care workflow in U.S. hospitals and it's been shown that they have created some unintended inequities for some patient groups,” says Dolatabadi.
“Embedded biases in health-care practices, reflected in the data used for model training, algorithmic decision-making, and the extent to which social determinants of health are considered in model design, can lead to unintended harm and exacerbate existing disparities. As health-care settings increasingly adopt these powerful AI models, now is the time to ensure they are responsible.”
The paper, Transitioning sleeping position detection in late pregnancy using computer vision from controlled to real-world settings: and observational study, was published in Scientific Reports.