By ‘Damola Adediji
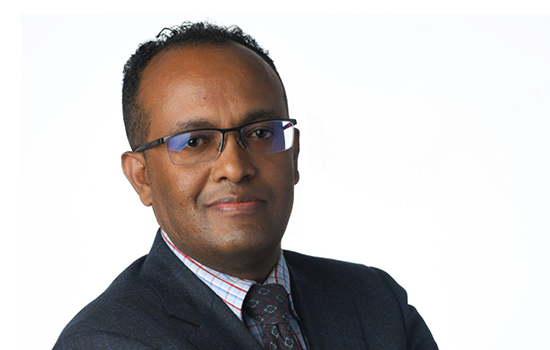
and IP Osgoode Affiliated Researcher
Artificial intelligence systems often “give the vibe” of complete automated processing without human involvement. However, as Dr. Tesh Dagne reminds us, upon a closer “vibe check” there are layers of unseen and under-appreciated human inputs, efforts, and labour involved. The efforts of those unseen human hands are, in fact, the engine of AI innovation.
Dr. Dagne is the Ontario Research Chair in Governing Artificial Intelligence and an Associate Professor at York University’s new Markham campus in the School of Public Policy & Administration. He also teaches Property Law at Osgoode Hall Law School, where he is an Affiliated Researcher with IP Osgoode. His current project, which he recently presented at the IP Scholars Africa conference at the University of Cape Town, highlights how copyright enables the proactive exploitation of digital workers’ contributions as inputs to AI training or, in some cases, AI-assisted outputs.
By bringing to the fore the roles of digital workers, Dagne hopes to unearth the collaborative creation that goes into the AI production chain and feeds into the AI output. His paper, “Unseen Hands, Invisible Rights: Unmasking Digital Workers in the Shadows of AI Innovation and Implications for the Future of Copyright Law”, is soon to be published in a forthcoming volume on IP’s Futures: Exploring the Global Landscape of Intellectual Property Law and Policy (Ottawa UP, 2025), which Dagne is co-editing with Alexandra Mogyoros and Graham Reynolds. His chapter probes the future of copyright law, attempting to turn the focus of copyright to collaborative authorship. This move, Dagne argues, could respond to demands for the fair allocation of rights between digital workers, as authors or joint authors in some cases, and AI designers as exploiters of digital works.
Digital Workers are the Lifeblood of AI Development
As Karen Hao puts it, “[AI] doesn’t run on magic pixie dust… [AI training] is a job that actually takes quite a bit of creativity, insight, and judgment.” Such ingenuity involves the preparation of data works for the datasets used to train and build AI technologies, which consists of a number of decisions as to the kind of data to collect, curate, clean, label, abstract, index, etc. The process of dataset development starts with formulating the problem, which is the conceptualization of the machine learning task by making the problems “into questions that data science can answer”. The task conceptualization is typically the responsibility of the AI designer, which may be an AI company like Open AI or Anthropic AI, for example, or platform company like Microsoft, Meta, or Amazon. After the conceptualization process comes the data collection, refining, and measuring stage. Dagne’s focus is on the “digital workers” who enter the picture at this stage in the AI production process.
According to Tubaro et al., these digital workers contribute to the training process of AI systems in three steps: generating and annotating data (AI preparation), verifying model output (AI verification), and directly mimicking model behaviour to produce a service (AI impersonation). They range “from higher-skilled, ‘macro-task’ […] workers [who] offer their services as graphic designers, computer programmers, statisticians, translators, and other professional services, to [those engaged in] ‘micro-task’ [work] which typically involve clerical tasks that can be completed quickly and require less specialized skills.” (Berg et al.) As described by C. Le Ludic et al, “complex projects are broken down into smaller, easily accomplished tasks, which can then be distributed to a large number of workers.” Micro-task activities mainly involve the AI preparation aspect of AI training processes but can also include the AI verification and AI impersonation steps in AI training.
The Copyright Question
Much of the debate around copyright and AI has focused on whether using the underlying work of which inputs are constituted (the images, texts, musical works and other subject matter) for unauthorized learning constitutes copyright infringement. However, Dagne’s focus is on the copyright that can subsist over collected data, as we see in some US and Canadian cases, and whether digital workers’ activities in the preparation of training data sets in the AI pipeline could itself give rise to a copyright interest. This question can be answered by examining the nature of digital workers’ contributions to the tasks assigned to them and the ownership of copyright under the contractual agreements that digital workers sign with platforms.
Digital workers in the AI production value chain collect raw data and help add extra meaning by associating each piece of data with relevant attributive tags. Although some have argued that this attributive task is a mundane exercise that could ultimately be automated, others like Ekbia and Nardi have contended that tasks such as attribution will always be assigned to humans because of their capacity to recognize and classify data. Indeed, human intervention is now in demand to recognize the nuances and sophisticated details of specific data. As noted by D’Agostino et al., an example of such demand is in the medical field, where an understanding of scientific vocabulary is required.
From a doctrinal perspective, the copyright question is whether the contribution of digital workers described above meets the threshold of originality—which is defined, in Canadian law, by the Supreme Court of Canada’s ruling in CCH, and requires more than trivial skill and judgment in the selection or arrangement of data. If so, we might ask whether recognizing the copyright status of such contributions could address these workers’ invisibility. Even if, on account of originality, the tasks executed by digital workers amount to authorship, of course such authorship does not automatically translate into ownership. The ownership of the creative tasks conducted by digital workers as part of the collaborative venture is determined either by the workers’ status as employees or otherwise by contract—which means that it is determined in the context of significant power asymmetries and the routine exploitation of digital workers.
If copyright entrenches the inequities of an asymmetrical situation—by ensuring that the collective effort of digital workers in compiling essential datasets for AI training and AI development remains unseen and undervalued—Dagne thinks the time has come to confront its complicity. He suggests that, spurred by the arrival of AI, the copyright system needs to restructure the relationship between authors-as-(data)workers and corporate proprietors in pursuit of greater fairness.
‘Damola Adediji is a Visiting Researcher with IP Osgoode and Doctoral Candidate with the Centre for Law, Technology & Society at the University of Ottawa.