In recent years, the rise of Industry 4.0 has accelerated the digitalization of the industry, blurring the boundaries between information technologies (IT) and operational technologies (OT). This convergence has brought about new challenges in cybersecurity, including emerging attack vectors, threats, and security vulnerabilities. To protect industrial infrastructure from these risks, monitoring and detection systems have become essential. However, these systems require diverse and high-quality datasets to effectively adapt to specific industrial systems. Unfortunately, acquiring such datasets from real-world factory setups is costly and challenging due to privacy and sensitivity concerns.
To address the lack of data, industrial testbeds have emerged as valuable resources. These testbeds, including simulated physical laboratory environments, virtual factories, honeynets, and honeypots, provide the necessary datasets for analyzing, testing, and evaluating cybersecurity solutions. The establishment of this infrastructure, using received CFI and ORF funds, at the Behavioral-Centric Cybersecurity Center (BCCC) in York University aims to revolutionize cybersecurity threat detection and prevention. The focus is on studying normal and abnormal cyber activities and patterns. Currently, the BCCC has four testbeds, including Honeynet, IoT transportation (CAV network), IoT and IIoT for Smart City, and Threat Hunting (including Malware Analysis and Attack Detection). Below are several of our ongoing research and development projects.
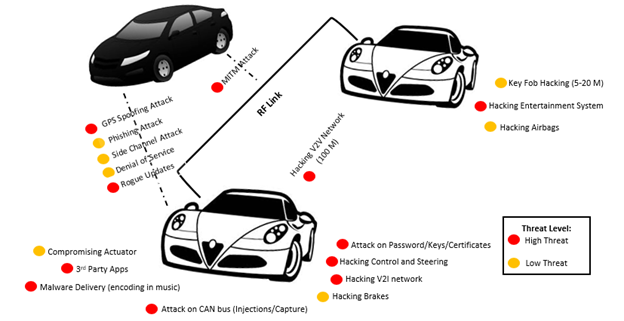
Transportation Network Security
The rise of connected and automated vehicles (CAVs) presents transformative opportunities for the automobile industry, yet certain obstacles must be overcome before widespread deployment is viable. Incorporating smart devices and communications into CAVs introduces critical cybersecurity risks and safety considerations. Unfortunately, these systems' interconnected nature and automation render them more susceptible to cyberattacks, including in-vehicle attacks and communication breaches. Consequently, new cybersecurity risks emerge. However, connectedness and automation make these systems more vulnerable and increase the consequences of cyberattacks, including in-vehicle attacks (e.g., hijacking attacks) and vehicle-to-everything communication attacks (e.g., data theft), introducing new cybersecurity risks. The consequences of a CAV being hacked could result in compromised features, resulting in accidents, damage, financial loss, personal injury, and even death. The objective of this R&D project is to design, develop and implement an intelligence-driven solution as a holistic countermeasure and evaluate the final solution in our CAV test-bed including more than 32 transportaiton network IIoT devices.
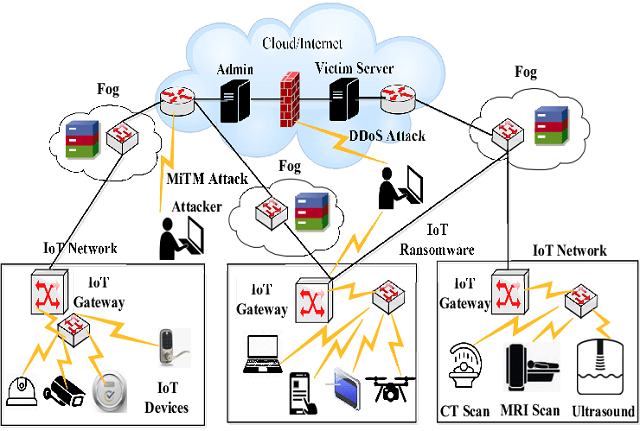
IoT and IIoT Network Security
In recent times, the increasing popularity of the Internet of Things (IoT) has opened up promising opportunities for the advancement of home automation systems and various industrial applications. This has led to the emergence of the Industrial Internet of Things (IIoT), bringing automation to industries. However, with these advancements, IoT faces numerous cybersecurity challenges requiring innovative approaches to ensure robust security. Furthermore, the security vulnerabilities associated with IIoT are even more concerning. This R&D project aims to address these challenges by conducting extensive research on cybersecurity threats and their management within IoT and IIoT network traffic. The insights gained from this research will be utilized to propose, design, and develop several intelligence-driven solutions that serves as a comprehensive countermeasure to mitigate these threats effectively. We will text and evaluate the final solutions in our IoI-IIoT testbeds including more than 75 Internet of Things devices and equipment.
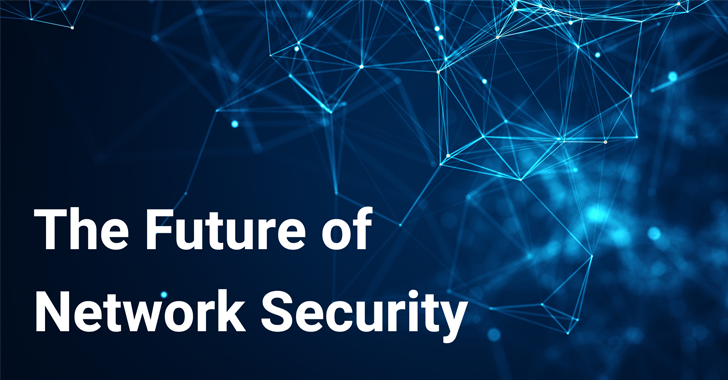
Universal Anomaly Profile (UAP)
Anomaly detection aims to find data activity patterns that do not conform to established and expected behaviours. Anomaly detection has many applications, such as detecting intrusion, fraud, data leakage, etc. The main idea in this project is to build a typical hierarchical profile for an organizations’ benign users, called a Universal User Profile (UUP), and a profile for users with behavioral anomalies, or “bad users”, a Universal Anomaly Profile (UAP). The proposed model will be called Universal Anomaly Detection (UAD) and, along with a human-centric infographic model, will contain multiple data visualization techniques to allow for improved visibility, faster troubleshooting, and streamlined onboarding. It will be developed as a new component for all available IT systems security management solutions, including Intrusion Detection and Prevention Systems (IDS/IPS), Unified Threat Management (UTM), Security Information and Event Management (SIEM), etc. and will be tested unsing our network security testbed including 50 VMs (covering different OSs and Users behaviours) and 11 servers (such as Web Server, email server, Database Server, DNS Server, File server, FTP server, DHCP servr, etc.).
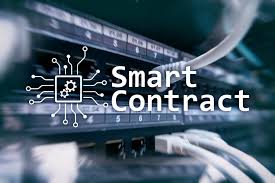
Smart Contracts Vulnerabilities Profiling
Smart Contracts are self-executing programs operating on blockchains like Ethereum, with a significant role in decentralized applications and cryptocurrency management. However, they are susceptible to attacks due to technical flaws in design and implementation. Smart Contracts enable automated and trustless agreements without intermediaries, and their immutability after deployment on the blockchain makes security considerations crucial during development. Nonetheless, recent devastating attacks and numerous vulnerabilities have raised concerns about the technology's sustainability. While Smart Contracts offer a powerful tool for developing decentralized applications, their implementation on blockchain platforms introduces security challenges stemming from programming vulnerabilities, which can lead to substantial economic consequences. This project aims to develop a Universal vulnerability profiling system for Smart Contracts to address these issues.
Partners:


Acknowledgment
We acknowledge the support of the Canada Foundation for Innovation (CFI) and Ontraio Research Fund (ORF).

