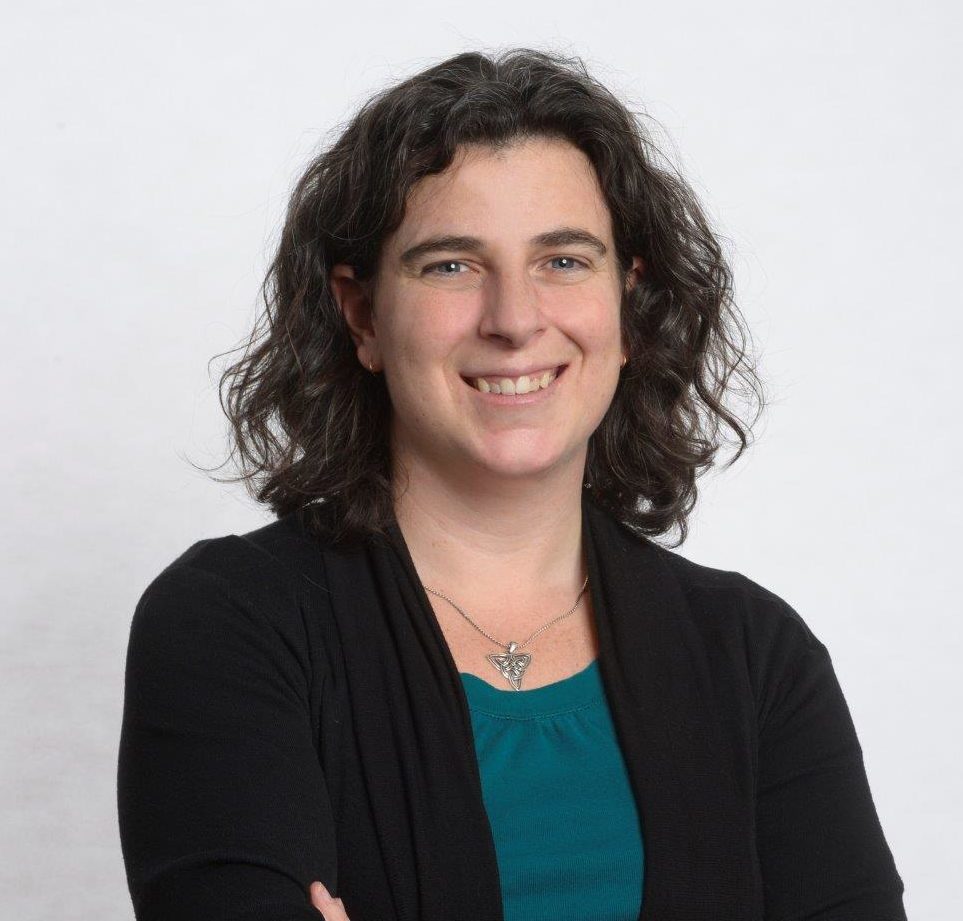
What level of immunity against COVID-19 do you have after being vaccinated or contracting the virus? Faculty of Science professor Jane Heffernan is receiving a $200,000, one-year grant from the National Research Council of Canada (NRC) to understand the rate of immunity in both of these scenarios.
The project is part of the NRC’s Pandemic Response Challenge program, designed to bring the best Canadian and international researchers together to fast-track research and development aimed at specific COVID-19 gaps and challenges as identified by Canada’s health experts.
Heffernan, Inaugural York Research Chair (Tier II), Multi-Scale Methods for Evidence-based Health Policy in the Faculty of Science, is leading the study with colleagues James Ooi, the NRC’s Pandemic Response Challenge program project lead, and M. Sajjad Ghaemi, NRC research officer, both from the NRC-Fields Collaboration Centre.
“Different vaccines elicit an immune response using different pathways, which affects the level and type of immunity you build,” says Heffernan of the Canadian Centre for Disease Modelling. “With this research, we’re tracking the activation of the immune response that’s been excited by vaccines, looking at the generation of antibodies, as well as memory B cells and T cells. Clinical trials can measure the number of antibodies, but they don’t measure B cells and T cells.”
To do this, the researchers will combine mathematical models of immunity development (mechanistic models) with machine learning algorithms to better understand the outcomes of immunity to the SARS-CoV-2 virus after one- and two-dose regimes of adenovirus (AstraZeneca, Johnson & Johnson), mRNA (Pfizer and Moderna) and protein subunit (Novavax) vaccines. They will model the effectiveness and immunity response to the virus, including pathogen mutations and variants, when vaccines doses are given days or weeks apart or, as is the case in Canada currently, four months apart.
The researchers hope the mechanistic models will enrich the dataset upon which the machine learning framework is trained. By combining new datasets that are being released publicly, this approach can potentially advance the accuracy of the machine learning framework. This will allow the researchers to classify outcomes of vaccinations as emerging evidence becomes available.
The idea is to uncover the complex interactions between interferon signalling pathways and the adaptive immune response to SARS-CoV-2 infection and vaccination.
“When you model outcomes in antibodies, it’s important to try to model the development of these memory cells in the background. Antibodies protect you from being infected and if they fail, it’s the memory cells that give you that activate factor that allows you to have a milder infection,” says Heffernan.
One of the goals of this research is to tailor vaccines to people’s body chemistry. “This is well into the future, but the goal eventually is to develop in-house models for mRNA, adenovirus and protein subunit vaccines that can be used to inform what type of vaccine a person should get depending on the characteristics of their immune system,” says Heffernan.
In the short term, the researchers hope to predict the outcomes in children of various vaccines, even without the results of a clinical trial. Based on the differences in immune response of children versus adults, the idea is to change the machine learning and mechanistic models calibrated for adults so that they fit the characteristics of children.
The modelling can also be expanded in the future to test other types of vaccines for COVID-19, in addition to vaccines for other viruses.
The data will be provided to public health agencies, such as the Public Health Agency of Canada, the National Advisory Committee on Immunization and the Canadian Immunization Research Network, and academic researchers to inform vaccine design and policy, and predict safety and efficacy of different vaccine types.