Steven Xiaogang Wang, a professor in York University’s Department of Mathematics & Statistics at the Faculty of Science, and a member of the Laboratory of Mathematical Parallel Systems, has had a U.S. patent approved for an algorithm that will reduce the training time of artificial intelligence (AI) machine learning (ML).
The patent, titled “Parallel Residual Neural Network Architecture and System and Method for Training a Residual Neural Network,” was inspired by a 2018 paper titled “Decoupling the Layers in Residual Network.” Both were based on collaborations with Ricky Fok, a former postdoctoral Fellow student; Aijun An, a professor in the Department of Engineering & Computer Science; and Zana Rashidi, a former graduate research assistant who carried out some of the computing experiments.
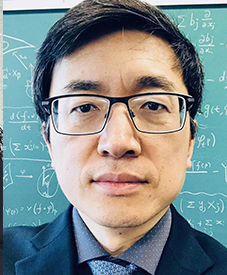
The now-patented algorithm, approved this year, was a result of six months of research at York. It was submitted to the United States Patent and Trademark Office in 2019. The algorithm’s framework is based on mathematical arguments that helps significantly reduce the training time of machine learning, as it absorbs, processes and analyzes new information. It does so by using a mathematical formula to allow residual networks – responsible for the training of AI – to compute in parallel to each other, thereby enabling faster simultaneous learning.
Wang’s desire to accelerate machine learning’s abilities is driven, in part, by a specific area of AI applications. “I want to apply all the algorithms I develop to health care,” Wang says. “This is my dream and mission.”
Wang has especially focused on using AI to improve care for seniors and that work has previously earned him the Queen Elizabeth II Platinum Jubilee Award from the House of Commons for initiatives during COVID-19 to mitigate the spread of the virus in long-term care facilities.
Wang plans to use the patented algorithm in ongoing projects that aim to provide smart monitoring of biological signals for seniors. For example, it could be used in long-term care to continuously monitor electrocardiogram signals at night to register heartbeats that have stopped. To move towards that goal, Wang is also working on building an AI platform that will complement those ambitions, and expects it to be ready in several years.
He is deeply invested in the social impact of AI as a member of the York organized research unit Centre for Artificial Intelligence & Society, where researchers at York who are collectively advancing the state of the art in the theory and practice of AI systems, governance and public policy.
“I can use the machine learning to help the long-term care facilities improve the quality of care, but also help out with the struggles of the Canadian health-care system,” says Wang.