With the recent advances in machine learning techniques, Joel Zylberberg, an associate professor in the Department of Physics and Astronomy at York University, decided it would be useful to science students to understand these methods, so he developed a graduate course to share his knowledge.
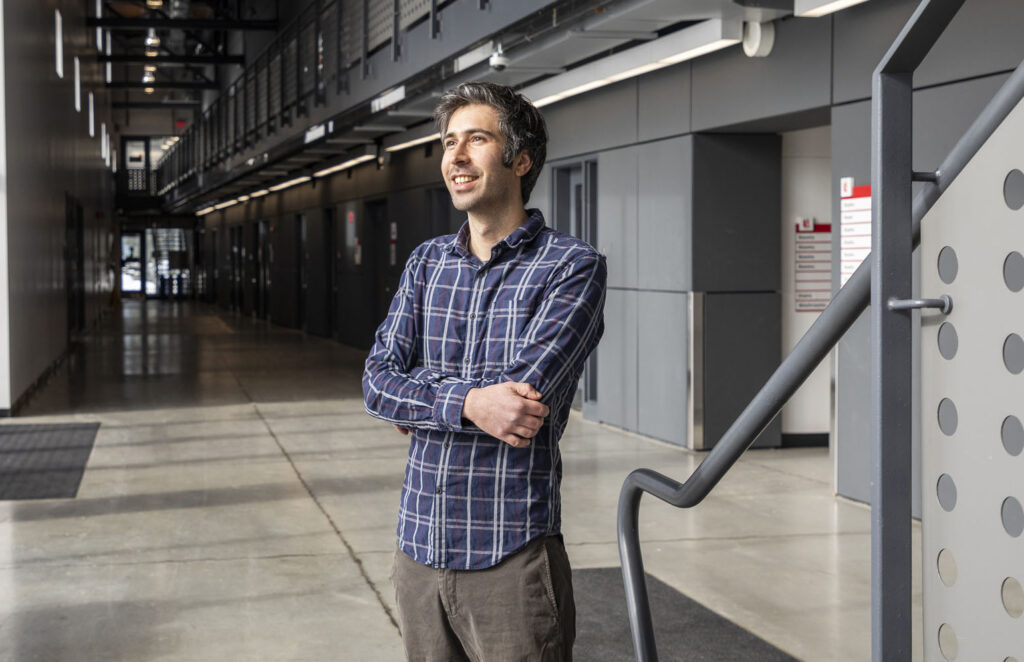
His course, Deep Learning for Physicists, made its debut in 2021 and was repeated in 2022. Its positive reception encouraged Zylberberg to think more broadly, and this year he is offering the course through the Fields Institute to graduate students from the 12 Ontario universities that are members of the institute.
“I like the idea of having more downstream impact from my teaching, working with a class of 35 rather than five,” Zylberberg said. “The applications are pretty diverse; students are working with everything from space science to quantum mechanics. I get to interact with all of this fun science through a set of methods that I know pretty well.”
He noted that the core machine learning ideas date back to the 1980s and 1990s, “but more sophisticated hardware now allows us to make models on a different scale.”
“Machine learning methods have come to dominate a lot of quantitative work and I wanted to give graduate students a strong foundation,” he continued. “Computer science students may have done a course or two in machine learning, but there’s no undergraduate course available for natural science students. Most of the students who enrol in the course have a pretty sophisticated mathematics background with solid undergraduate training in calculus. About half of them now have some prior machine learning experience and come to the course to learn to think about this topic in a more systematic way.”
The first half of the course is devoted to understanding the theory of why systems are built in a particular way and how they work; the second half of the course focuses on applying the theory. The assignments require students to make various machine learning applications and their final project asks them to solve a scientific problem using the course methods.
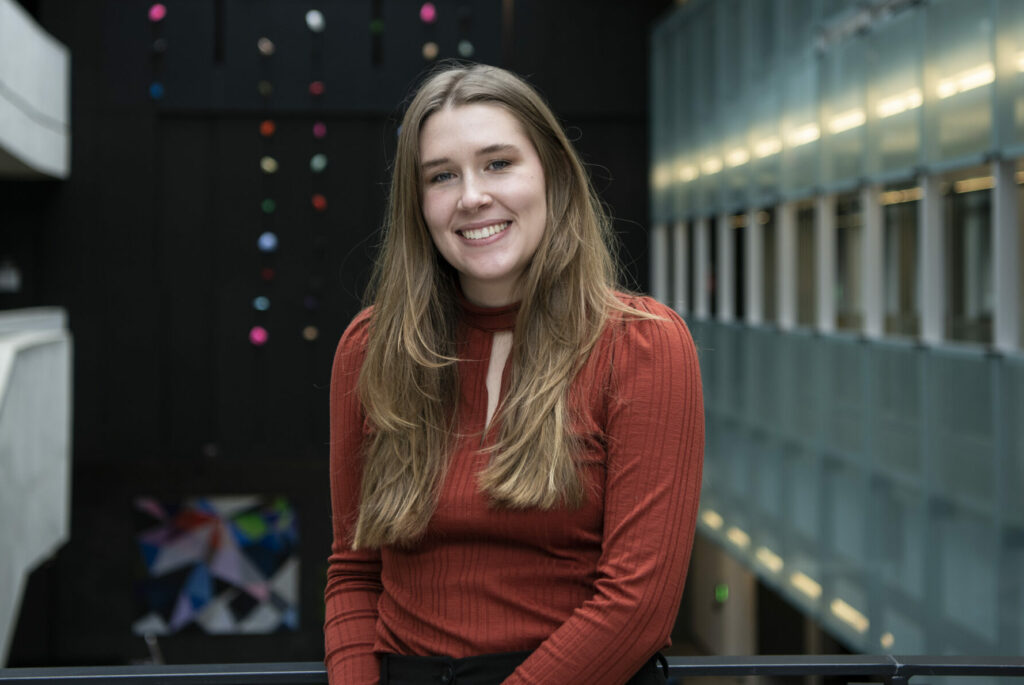
Jordan Krywonos, a York PhD student in cosmology, took the course last year and now serves as a teaching assistant for the course.
“My supervisor had an idea for a project that involved machine learning, but neither of us had experience with these methods,” said Krywonos, who is based at the Perimeter Institute in Waterloo, Ont. “It was a good opportunity to have expert guidance in how to solve any machine learning problems we encountered.”
She applied machine learning to her work in predicting the dark matter halos for a population of galaxies, a project Krywonos scaled up afterward.
“I continue to use the course techniques for this ongoing project,” she said. “I’m trying various machine learning algorithms to see which one has the best performance.”
In another example, one of the graduate students in Zylberberg’s lab found a way to use machine learning to see glowing cells in a mouse’s brain more clearly by using a model that removed much of the data noise from the measuring device, improving the signal-to-noise ratio by a factor of 20.
During the course, Zylberberg has the class review research papers together so they can analyze the machine learning applications employed in each study. For Krywonos, it has been helpful, since machine learning is being used more frequently in cosmology today.
“When I read through a research paper now, I can understand and analyze it better,” she said.
This year’s class is being taught in hyflex fashion: all of the students, whether at York or at other universities, have the option of coming to class in-person or joining via Zoom. The online option allows students across Ontario to enrol in a valuable course to which they wouldn’t have access otherwise, while the in-person option provides students with the opportunity to enjoy a traditional classroom setting. No matter which option they choose, Zylberberg aims to provide them with an equitable experience.
“We’ve gotten a lot of practice using hybrid formats over the last few years, given the pandemic,” Zylberberg said.
The final few sessions of the class are devoted to a mini-conference where the students present their projects, honing skills that will be useful in the workplace.
“Scientists need to be able to communicate what they are doing and why,” Zylberberg said. “It’s a broadly useful skill in academic research, as well as in industry where one might be selling a product or pitching a new venture to investors.”
Cheryl van Daalen Smith, FGS associate dean, academic said, “With its relevant subject matter, its accessible delivery mode and its broad reach, Deep Learning for Physicists is an example of the innovative graduate courses that the Faculty of Graduate Studies is proud to offer.”