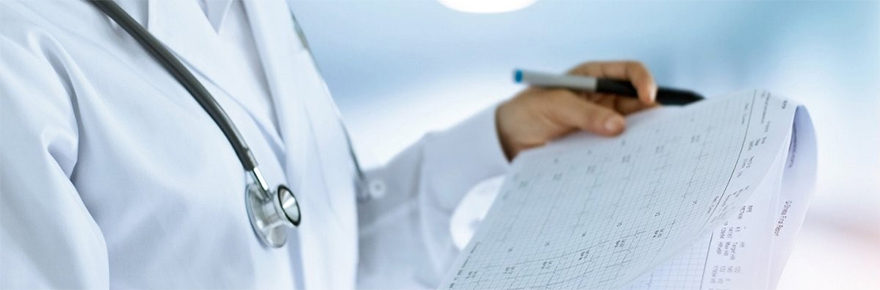
A new study out of the Lassonde School of Engineering and Sunnybrook Hospital offers the potential for doctors to personalize cancer treatment.
Breast cancer will affect roughly one in eight women at some point in their lives, and it kills about 5,000 women every year in Canada. Among the available treatment options, chemotherapy is the most common and offers great hope of saving lives.
Unfortunately, 30 to 40 per cent of patients do not see improvements to their prognoses following chemotherapy, a treatment that comes with intense side effects. And, what’s more, doctors must wait until a patient’s chemotherapy regimen is well advanced, or even concluded, before they can know with any certainty whether the treatment has worked.
A new study involving researchers from the Lassonde School of Engineering and Sunnybrook Health Sciences Centre offers a potential solution to this clinical problem.
The study, which is published last week in Nature’s journal Scientific Reports, combines state-of-the-art imaging techniques with sophisticated machine learning using artificial intelligence (AI). The result is a methodology that could allow clinicians to predict – before or soon after treatment begins – how well individual breast-cancer patients will respond to chemotherapy.
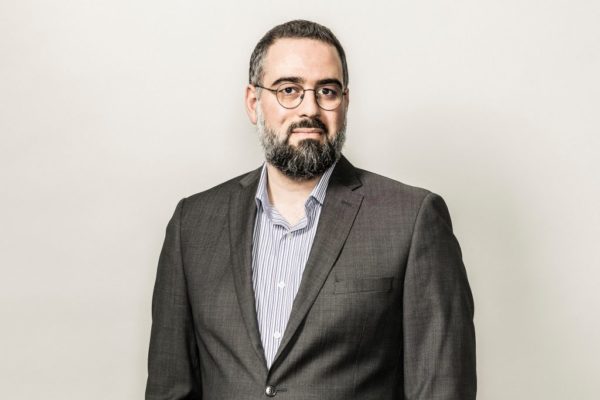
“Currently, there is no clinical method to predict the response of breast cancer patients to chemotherapy,” says the study’s lead author, Ali Sadeghi-Naini, an associate professor and York Research Chair in the Department of Electrical Engineering and Computer Science at the Lassonde School of Engineering. “But in this paper, we demonstrated how an AI architecture can be trained for deep machine learning of images to predict the response of patients to chemotherapy before the patient has started the treatment.”
For breast cancer patients, the implications are immense. The study, which drew on existing clinical data, includes 10-year survival analyses that demonstrate significant differences in survival rates between those patients that the study’s prediction model identified as ‘responders’ to chemotherapy and those identified as ‘non-responders.’
The current standard method for evaluating patient response to chemotherapy is measuring changes in tumour size after the conclusion of treatment.
“Generally, when someone receives chemo, what happens is three to five months after chemo, a CT or MRI scan will be done,” says co-author Dr. Gregory Czarnota, chief of the Department of Radiation Oncology, Sunnybrook Health Sciences Centre. “Size is measured at that point. By then, you’ve lost the opportunity to do something about it. You can’t alter the treatment or tailor it to the patient’s individual response.
“So, if we can tell with near 90 per cent accuracy, using this methodology we’ve developed, that a person won’t respond to chemo, there’s no reason to go forward. We could also tailor treatments according to patient’s predicted level of response. We could alter drug types, alter dosages, or change the length of a treatment regime. We could also consider alternative treatment routes for patients who are predicted not to respond at all to chemo.”
The study’s methodology involves an artificial intelligence program designed to learn how to make minute distinctions between Quantitative Ultrasound (QUS) parametric images of cancerous tumours, at a volume and level of detail that would be impossible for human beings. The new study builds on previous research on QUS parametric imaging, an advanced technology that transforms raw ultrasound signals (which are often discarded by the scanner after generating conventional images) to measure and visualize biophysical properties of tissues.
In a prior paper, published last year, Sadeghi-Naini, Czarnota and their co-authors demonstrated QUS parametric images could be used to distinguish between individual tumours in terms of their aggressiveness and responsiveness to chemotherapy.
“In this new study, we integrated AI and QUS imaging to make better use of the two,” says Sadeghi-Naini.
The researcher focuses on Locally Advanced Breast Cancer (LABC), a particularly virulent and relapse-prone form of the disease that is marked by especially large tumours. Up to 20 per cent of breast cancer cases are LABC.
The study was led by Sadeghi-Naini, along with graduate students Hamidreza Taleghamar and Seyed Ali Jalalifar. It was co-led by Czarnota, who is also a clinician scientist at the Sunnybrook Research Institute.
The study was funded by Ontario’s Ministry of Colleges and Universities through the Early Researcher Awards (ERA) program, the Natural Sciences and Engineering Research Council (NSERC) of Canada, Canadian Institutes for Health Research (CIHR), and Terry Fox Foundation through a New Frontiers Program Project Grant.